✨Guide: Ethically Using AI with Student Data
Canvassing options for professors to use AI without risking student data.

[image created with Dall-E 3 via ChatGPT Plus]
Welcome to AutomatED: the newsletter on how to teach better with tech.
Each week, I share what I have learned — and am learning — about AI and tech in the university classroom. What works, what doesn't, and why.
In this fortnight’s Premium edition, I present a guide for how to ethically use student data with AI tools.

With the rapid advancement of AI technologies, there is a pressing need to harness these tools responsibly, especially when it comes to the privacy and security of student data. The integration of large language models (LLMs) like ChatGPT, Gemini, and Claude into the educational sphere offers unprecedented opportunities for personalized and responsive pedagogy. However, these opportunities come with significant privacy challenges.
The utility of AI tools is contingent on their access to large volumes of high-quality data — data that, in many contexts, includes personally identifiable or otherwise protected student data. This requirement raises significant concerns about data security, as data breaches or misuse can lead to privacy violations.
Addressing these privacy issues requires more than just technological safeguards; it demands a comprehensive approach involving knowledge, expertise, and deliberate action. Educators must become adept not only at using AI tools but also at understanding the implications of their use, especially when it comes to student data privacy.
In this Guide, I canvas the option space and offer considerations related to each of the seven main options that I outline.
Table of Contents
🛑 Option 1: Don’t Run Student Data
Through AI At All
One strategy would be to limit AI usage strictly to tasks that do not involve student data, such as lesson planning, transcription of faculty meetings, brainstorming sessions, or providing generalized feedback on assessments like a mentor would. This approach would completely avoid the use of any AI tool with student data.
However, there are compelling reasons to not select this option.
Firstly, restricting AI tools in this way would significantly limit the pedagogical benefits that these technologies bring, particularly in personalizing education. AI's capacity to tailor instruction, assessment, and communication to the unique needs of individual students is akin to having a classroom full of skilled tutors for each student. The potential to enhance educational outcomes through such personalization is substantial, and forsaking these advantages could be seen as a step back from leveraging technology to improve education.
Secondly, the practicality of completely segregating AI tools from student data is increasingly unlikely, given the integrated nature of modern educational ecosystems. Most educational institutions, not just in the U.S. but globally, rely heavily on platforms like Google Workspace and Microsoft 365 for their essential computing needs, including email and document management. Even though users must opt-in to integrate these platforms with advanced AI functionalities — such as Google's Gemini or Microsoft's 365 Copilot — these ecosystems already process vast amounts of student data.
It's important to clarify that the argument here is not for a carte blanche approach where student data is indiscriminately processed through any AI tool available. Indeed, there may be specific AI applications and types of student data that should not be combined, based on the sensitivity of the data and other critical considerations that will be explored later in this guide.
Rather, the crux of the argument is that any decision to exclude student data from AI processing should align with your views on your use of your institution’s broader IT strategies. Since my view is that platforms like Google Workspace and Microsoft 365 are sufficiently secure in handling student data, my view is that the integration of Gemini with the former and Copilot with the latter doesn’t move the needle enough to justify not using those AI tools with student data.
In other words, if there is a concern about using Google's Gemini with certain student data, the same concern should logically apply to other Google services like Gmail or Google Docs, unless there is a principled reason to distinguish these services that does not compromise the operational integrity of the institution's reliance on these ecosystems. What, then, would be a principled reason to differentiate the use of Gemini from, say, Gmail, if both are part of the same trusted platform?
🦺 Option 2: Limit Data Use to
“Completely Safe” Categories
Another strategy would be for professors to be extremely selective about what sort of student data they run through AI tools. The goal would be to select only “completely safe” categories of data for exposure to the relevant AI tools.
What qualifies as “completely safe”?
It is hard to give a simple and general answer to this question because of the many different contexts in which professors find themselves. However, I think good frameworks for thinking about how each of us should answer this question are provided by relatively comprehensive laws that attempt to govern the use of student data, like the US federal law FERPA. (Other examples include the European Union’s GDPR.) These laws provide insight into many of the relevant sorts of considerations in defining the safety of various categories of data.
The core idea behind FERPA is that, unless a student who is at least 18 years old explicitly consents in writing to release or share their “personally identifiable” data beyond the relevant parties in their educational institution for legitimate educational purposes, it must not be released or shared, except for a relatively small swathe of special cases (e.g., for certain student aid purposes or in a safety-related emergency).
Personally identifiable data, in turn, is data that could reveal the identity of the student associated with it, either in a single instance or in a sequence of disclosures, when combined with other plausibly available information or background context.
A two-column spreadsheet titled “Student 03902” with grades paired with assignment names is not necessarily personally identifiable. On its own, it does not identify Student 03902. Nonetheless, it would be actually personally identifiable, if the spreadsheet could be associated, via other plausibly available information, with Student 03902. For example, if it is public information that Student 03902 is me because, say, 03902 is my university-assigned and public student ID number, then the student data on my grades in the spreadsheet is personally identifiable.
For my purposes here, I think a good starting point for defining or distinguishing completely safe student data is as data that is not personally identifiable even in principle. That is, it is not possible to combine completely safe data with other plausibly available information or background to identify which student it belongs or relates to.
A paradigm of completely safe student data would be sufficiently aggregated student data — that is, data that has been created by combining and generalizing from individual bits of student data, such that there is no way to infer from the aggregated numbers about any individual student’s data.
So, with this background in hand, let us ask: should we take the position that we only use completely safe student data with AI tools?
While there are benefits of this option that are not available to someone who takes Option 1 — for instance, sufficiently aggregated student data can still be useful for a variety of professorial tasks, like lesson planning or assessment analysis — my view is that it suffers from the same worries facing Option 1. Student data that is not completely safe is incredibly useful to run through AI tools, and it also already is running through the ecosystems operated by the same companies running some of the primary AI tools (like Google’s Workspace and Gemini). Again, the cost is too large and it is not feasible.
Note: While discussing the use of AI tools like Google Workspace's Gemini or Microsoft 365's Copilot, I have been assuming these platforms comply with privacy laws like FERPA and GDPR. This assumption rests on their established compliance mechanisms and regulatory oversight. However, given the rapid evolution of AI technologies and regulatory frameworks, educational institutions must, to some degree, actively verify these tools adhere to up-to-date data protection standards. This proactive approach ensures the ongoing privacy and security of student data.
🏰 Option 3: Stay Within Your Ecosystem
A third strategy for managing student data privacy with AI tools is to remain strictly within the confines of your institution’s established technological ecosystem, whether that’s Google Workspace using Gemini or Microsoft with Microsoft Copilot or Copilot for Microsoft 365.
This position advocates for a stance where any and all student data available within the institution's ecosystem — including those that may not be classified as "completely safe" (as defined above) — can be utilized for educational purposes through AI tools. While more restrictive approaches can be adopted based on specific needs or concerns, they often limit the pedagogical potential of AI. By fully embracing the capabilities of their ecosystem's AI tools, educators can significantly enhance the educational experience without compromising on data security, ensuring that student privacy is maintained under the umbrella of their institution’s compliance and security protocols. This approach capitalizes on the robust security and compliance frameworks these platforms are built upon, which are designed to align with stringent privacy laws like FERPA and GDPR.
However, this approach does come with certain limitations, particularly for professors who wish to explore innovative AI applications that extend beyond the confines of their institution's ecosystem. By restricting AI tool usage to only those integrated within platforms like Google Workspace or Microsoft 365, educators might miss out on specialized AI functionalities offered by external tools.
For example, certain AI tools developed outside these ecosystems might offer unique features for analyzing anonymized data sets or conducting more nuanced sentiment analysis, which are not yet available within the standard institutional offerings. Additionally, professors interested in customizing AI tools or integrating them with consented student data for specific research projects might find this approach overly restrictive, as it limits their ability to harness the full potential of emerging AI technologies.

🗣️ Option 4: Change the Consent Paradigm
Another option would be to get explicit written consent from students in order to use any of their data with AI tools.
In my experiences discussing with students whether they consent to me using their data, they always appreciate being asked, even if the data and use case are completely safe (and not covered by FERPA). Why? They tell me that they know that the university and their professors have access to so much of their data, but that they wish it were more transparent how it is being used. They appreciate being respected and included in the process of teaching them and assessing their work.
Given that I think that this general desire amongst students for greater data transparency is more than reasonable, it is my view that it is worth trying to respect. In general, professors should be honest and they should be in the position to convince (reasonable) students that their uses of student data are worthwhile and worth consenting to.
But the present question is the consideration of the option where professors seek to get consent for all uses of student data with AI tools, regardless of the use case or regardless of the nature of the data (e.g., whether or not it is completely safe).
It is a way to address the problem via a blanket strategy of informing students of how exactly we plan to use their data in our uses of AI tools and then getting consent about precisely those use cases. It requires changing the paradigm around consent — the default stance of the professor becomes one of consent seeking rather than one of avoiding scenarios that would require consent.
Should we take this option?
As I suggest when considering further options below, my view is that relying completely on consent — whether the data in question is completely safe, personally identifiable, or whatever — is too extreme.
For me, the clearest exception cases to such a broad blanket policy are uses of student data that are completely safe (not even in principle personally identifiable) where it is costly or inconvenient to get consent and there are serious benefits of me running such data through AI tools.
The next clearest are cases where the data is personally identifiable in principle, but where I can take reliable steps to make it not personally identifiable in actuality (e.g., anonymize it, per the below) and where it is stored in an ecosystem I am already relying on to protect personally identifiable student data like sensitive emails. And I would even go so far as to suggest that consent is not morally required or needed in cases where I am using AI tools in such an ecosystem on data that is personally identifiable.
The less clear cases are cases where the AI tools are third-party like Fireflies.ai, Otter.ai, Zapier, or Make. Even if the relevant data is not personally identifiable in actuality, these are cases where consent should be sought because of the increased risk, the expectation of students to not have their data used this way (or their lack of expectation that it would be used this way), and so on. I will discuss this sort of mixed or combination strategy below in the discussion of Option 7…
(This is to set aside the question of conformity to my university’s data classification and usage policies, which are somewhat idiosyncratic. Professors should be aware of those institutional policies with which they must be compliant.)
Note: When seeking student consent for using their data with AI tools, we must recognize and address the challenges of ensuring genuinely informed consent. The complexity of AI technologies, coupled with the intricate implications of data usage, may not be readily understood by all students. I have found this to be true in my own experimentation with consent-based solutions at my institution.
For instance, a student might consent to their data being used for 'enhancing learning algorithms,' not fully realizing this could entail detailed analysis of their interaction patterns and potentially sensitive performance metrics.
To tackle this, educators should provide clear, comprehensive explanations of what data is being used, how it is being processed, and what the outcomes might be. This could involve simplified briefings, examples of data use cases, or even Q&A sessions where students can express concerns and request further information. Ensuring that consent is truly informed not only respects student autonomy but also fortifies trust in educational uses of technology, making it crucial to invest time and resources in educational initiatives that enhance understanding of AI's role and impact in the learning environment.
🥷 Option 5: Pseudonymize or Anonymize
A fifth strategy would be for professors to pseudonymize or anonymize the student data before running it through the AI tools.
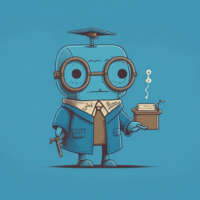
Subscribe to Premium to read the rest.
Become a paying subscriber of AutomatED to get access to this post and other perks.
Already a paying subscriber? Sign In.
A subscription gets you:
- • Access to All Tutorials and Guides
- • Two New Premium Pieces per Month
- • Free Access to Monthly Webinars
- • Access to Exclusive AI Tools
- • Discounts on Courses
- • One Free 1-Hour Consultation with Dr. Clay