Ease End-of-Term Headaches with AI
Plus, readers respond to my reflection on AI literacy last week.

[image created with Dall-E 3 via ChatGPT Plus]
Welcome to AutomatED: the newsletter on how to teach better with tech.
In each edition, I share what I have learned — and am learning — about AI and tech in the university classroom. What works, what doesn't, and why.
In this week’s piece, I…
share 3 easy ways to use AI to make the end of the semester hurt less
reflect on last week’s reflection on AI literacy (and share your responses to the poll)
explain which topics institutions request that I speak to them about (note: January’s booked solid)
and explain why I’m excited about Google’s now-available LearnLM
Let’s dive in!
Table of Contents

🧰 3 AI Use Cases for Your Toolbox:
Semester’s End Edition
For Accelerating Grading and Feedback
At the end of the year, it’s rewarding to see how far your students have come.
However, it’s very draining to grade and give feedback at scale, especially if you have a backlog by this time in the semester/term (and a lot of students).
AI can help you grade faster while maintaining quality. You can sign up for my upcoming December 6th webinar on AI-assisted feedback to learn a range of strategies, or try the one I explained in my spring piece on how I use ChatGPT to grade 2-3x faster.
For the latter, you use an LLM to convert your rough notes on each student’s work into polished ones in a target format; you focus on content, the LLM focuses on presentation. By only inputting your own notes, not student work, you maintain student data privacy.
You can kick it up a notch by creating a custom GPT or Google Gem for the purpose. (By the way, as of a recent update, you can now upload up to 10 files to each Gem, giving them additional context to provide more tailored and helpful responses.)
✨Relevant Premium Resources:
GPT: Educational Feedback Accelerator (pre-built GPT for this purpose)
Tutorial: How to Build (Better) Custom GPTs
Tutorial: Grading Handwritten Multiple-Choice Quizzes with ChatGPT Vision
Guide: How to Ethically Use AI with Student Data
For Making Office Hours More Productive
I find that end-of-term office hours often involve answering the same questions repeatedly. There is convergence in the content that my students are confused about, and there is convergence in how they ask about it.
Although you could address this issue with whole-class “office hours” that take place during normal teaching periods, there are downsides to that approach: you lose invaluable instructional time, students often haven’t thought enough about what they are curious about, they are shy in front of their peers, etc.
Here's how to use AI to enhance student meetings, which are typically one-on-one, so that they fill this gap and are more efficient for everyone:
Make office hours virtual
Record your office hours sessions using your institutional or preferred virtual meeting platform (e.g. Zoom, Microsoft Teams, or Google Meet)
Use your platform's built-in AI features — like Zoom AI Companion — to generate detailed transcripts from the recording, or use a data-safe LLM to do the same
Use another data-safe LLM like your institution’s Microsoft Copilot or Gemini for Workspace to extract and anonymize key Q&A pairs from these transcripts
Copy/paste these anonymized Q&A pairs into a shared cloud-hosted Google Doc or Word document for all students to reference
You can also make viewing this document a precondition on accessing your office hours sign-up link on, e.g., Calendly, if you use one
This approach respects student privacy while avoiding duplicate questions and saving everyone time — and helping those who couldn't or don’t want to attend.
✨Relevant Premium Resources:
Tutorial: How to Integrate Zoom AI Companion with Google Workspace
Tutorial: How to Integrate Zoom AI Companion with Microsoft 365
Tutorial: How to Run Open-Source Local LLMs on Your PC for Privacy
Guide: How to Ethically Use AI with Student Data
For Analyzing Areas for Review
End-of-term review sessions are more effective when guided by data.
Using the methods from my free newsletter from June on how ChatGPT’s Advanced Data Analysis tool democratizes data analysis for educators, you can analyze patterns in your anonymized gradebook before final exams or papers in order to address gaps in your students’ understanding.
One option would be to upload your anonymized current grade data and ask the AI to analyze statistically significant correlations between different assignments to identify weaknesses in how you scaffolded to end-of-semester summative assignments. You can then recognize which formative assignments — intended to prepare students and inform you about their understanding — fell short in their desired effect and need to be remediated in review.
Or, for targeted interventions, you could upload the same file and look for individual student performances that are significantly worse, statistically, than those of their peers.
Or you could do the same with assignments themselves, in order to inspect their content for areas where the whole class slipped.
This approach helps you prioritize review topics and target specific concepts where students need additional support.
✨Relevant Premium Resources:
🤖 AutomatED Updates
📝✅ December 6th Webinar:
On Feedback & Assessment
Given the positive response to our recent webinars, I will be hosting one final Zoom webinar for the year. The topic: accelerating and improving feedback with AI.
You can check out the dedicated webinar webpage for more detail or sign up directly below, but the following bullet points convey the highlights.
Dates and Numbers
Location, Date, Time: Zoom on Friday, December 6th from 12pm to 1:30pm EST
Standard Price: $150
Early Registration Price: $75
✨Premium Subscribers’ Price: $60 (discount visible on webinar page for logged in users)
Early Registration Deadline: Monday, December 2nd at 11:59pm
Total Available Seats: 50
Minimum Participation: 20 registrations by Monday, December 2nd; if we do not reach 20 registrations by this date, all early registrations will be fully refunded and the webinar will be canceled/rescheduled
Money-Back Guarantee: You can get a full refund up to 30 days after the webinar’s date, for any reason whatsoever
What To Expect
Live 90-Minute Interactive Webinar on Zoom:
Framework for evaluating when and how to use AI in feedback
Live demonstrations of feedback generation with ChatGPT, custom GPTs, and Claude
Practical strategies for maintaining student data privacy
Concrete examples of using LLMs as mentors, student simulations, and teaching assistants
Extended ethics discussion and Q&A session (with me, Dr. Graham Clay)
High-Value Post-Webinar Resources:
Complete video recording, AI-generated summary, and presentation slides
Three complimentary ✨Premium pieces:
Curated collection of feedback-specific prompts for common assignment types
🧪 Beta Test Next Week:
AI Course Scheduler
The beta version of my AI department course scheduler is now ready for trials, with beta participants being contacted with details next week.
The tool accepts your existing faculty preference spreadsheets and scheduling data — no need to change your current data collection process — and then uses AI to interpret and analyze this information like a human scheduler would. The ultimate result is faculty-to-course assignments (and optional room scheduling), all while respecting and optimizing within your constraints.
Interested? Email me to:
Join beta testing to receive a discounted rate going forward
Get notified when it's publicly available
Learn more about pricing and features
Know someone who handles scheduling? Please share this with them!
Consultations and Presentations
My January external webinar/presentation calendar is now full with 6 sessions booked at institutions around the globe.
Popular sessions include big-picture primers to get whole departments or institutions up to speed on AI pedagogy, deep dives on custom GPTs (or Gems) and how to build a community of practice around them, and training students to use AI. (Not to mention the ever-popular AI misuse prevention talk.)
Limited February and March slots remain for these sessions, as well as for one-on-one AI pedagogy consultations and custom AI workflow development.
Interested in one of these? Feel free to schedule a free 30-minute exploratory call or email me:

✉️ What You, Our Subscribers, Are Saying
On AI Literacy
In my “Idea of the Week” last week, I reflected on a paradox I encountered while building an AI-powered faculty-to-course scheduler: while it seems you'd need deep technical knowledge to effectively use AI for complex tasks, sometimes the reverse is true. I argued that with sophisticated enough prompting skills and broad domain understanding, you can often acquire technical knowledge along the way through AI itself.
This challenged my previous stance that field-specific expertise was always necessary for effective AI use. (See here for a free piece on this topic, and see here for a ✨Premium deep dive on it.) I explored how this "prompting paradox" might suggest a new kind of technical literacy — one more focused on problem decomposition and AI communication/management than traditional technical skills.
In a poll, I asked you to weigh in on what AI literacy requires, offering options ranging from prompting ability (option A) to pre-existing domain expertise (B) to an understanding of AI itself (C) and of AI ethics (D).
While my “Idea of the Week” expressed a shift toward emphasizing prompting skills over pre-existing domain knowledge, I want to note that two factors shaped my evolving view. These have become clearer to me after thinking about this topic more.
First, my course scheduler project benefits from clear empirical testing at each stage — I can verify if the AI-generated code works, whether there are errors, etc. without needing deep programming expertise. This isn't true in many other contexts where external validation isn't available (in a timely fashion, at least), making pre-existing knowledge more crucial.
Second, as AI systems become increasingly reliable at producing high-quality outputs regardless of prompting approach, the importance of pre-existing domain expertise may diminish. Just as we need less technical oversight when working with highly competent human specialists, the game changes when AI reliability reaches a certain threshold. AI can complete relatively straightforward coding tasks with great reliability already. This may have been skewing my thinking.
Anyway, without further ado, here are the results of the poll:
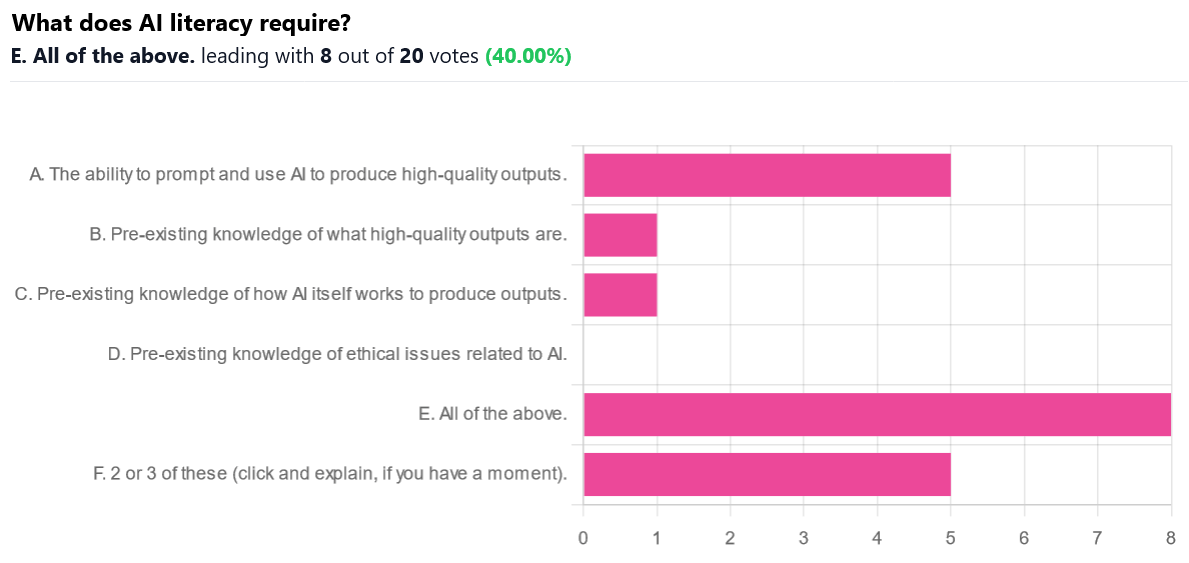
Here are some of your comments:
“Quite a bit is included in or implied by choice A!”
“Great reflection! AI literacy is a brand new concept since ChatGPT broke in and reached everyone, everywhere. Before that, it was in the [realm] of computer science and related areas. Now we wonder what AI skills (aka AI literacy) should every worker person should know (or recommended), and moreover, what AI skills should educators teach students. What is AI literacy? What does it comprise?
You probably hit the right keys in your survey. I guess all of the options have some merit to be included as AI literacy. However, if I had to prioritize them, I would highlight a) (ability to prompt) and b) (pre-existing domain knowledge). To me, option c) is somewhat related to a): good prompting comes when you know how AI works. Or at least, it helps a lot.
Coming back to your question (whether to teach traditional technical skills or advanced prompting), I feel like the second must have a spot in our syllabus. As you suggest, some basic knowledge in any domain can help you use good prompting (AI literacy) to solve problems (and probably, acquire new knowledge along the way), as long as you also have good critical thinking skills.
At least, this is my experience this semester, showing my students in class how to use a few simple prompts to learn (accounting) new content. At this point, my only goal is to show them AI is a great tool to help them learn. Haven't really got any further at this regard. Working also on how to prompt AI to produce high quality multiple choice tests. A big thank you for continuing sharing your thoughts and reflections in this rapidly changing AI+Education world.”
“I think you need a combination of A and B. You need to know the basics of good prompting along with the ability to critically evaluate the outputs to avoid some of the common pitfalls of GenAI (bias, hallucinations, and incompleteness).”
Feel free to share your thoughts if you didn’t get a chance to do so last week:
👀 Google’s LearnLM is Here
Google has released LearnLM, an experimental task-specific model fine-tuned to align with learning science principles, for all to access. Available for free through Google AI Studio, Google claims LearnLM excels at inspiring active learning, managing cognitive load, adapting to learners, stimulating curiosity, and deepening metacognition. The model comes with pre-built system instructions for various educational tasks like test prep, concept teaching, text releveling, guided learning activities, and homework help. Each instruction set is carefully designed to implement specific learning science principles while maintaining engagement and effectiveness.

Model selection options in Google AI studio.
From my perspective, the challenge for (higher) educators lies in getting LearnLM into students' hands via user-friendly interfaces. While students can access it directly through Google AI Studio, this isn't ideal for structured learning environments. I’ve written a lot about how Google AI Studio can be used by educators for uses like quiz creation and writing grant applications, but LearnLM is a model that has its greatest potential when used by the students themselves.
I think the most promising deployment options would be through Google Gems (if Google enables LearnLM as a model choice) or via API integration with other educational tools like custom GPTs.
I am exploring ways to easily deploy LearnLM for student use in a future ✨Premium Tutorial. It would be nice to deploy LearnLM to create adaptive homework help systems, build scaffolded writing assistants that grow with student ability, develop interactive exam prep tools, or … .
More soon!
What'd you think of today's newsletter? |

![]() Graham | Let's transform learning together. If you would like to consult with me or have me present to your team, discussing options is the first step: Feel free to connect on LinkedIN, too! |